论文基本信息
item | value |
---|
title | Sat2Scene: 3D Urban Scene Generation from Satellite Images with Diffusion |
publication | CVPR 2024 |
group | 1 ETH Z¨urich 2 The University of Tokyo 3 Zhejiang University 4 Microsoft 5 University of Amsterdam |
link | https://arxiv.org/abs/2401.10786 |
1 sentence description | 写作很好,但是个人认为很难说是一个亮眼的工作 |
论文创新点
The contributions of this paper are three-fold:
- We present a novel diffusion-based framework Sat2Scene for direct 3D scene generation, which is able to generate 3D urban scenes just from satellite images. (对做了什么的描述)
- To ensure consistent image generation from any view, a novel diffusion model with sparse representations is proposed to generate scene features tightly associated with the geometry directly in 3D space. To the best of our knowledge, we are the first to combine diffusion models with 3D sparse representations. (第一个使用diffusion+sparse 3d representation)
- Our model demonstrates the capability to produce photorealistic street-view image sequences with robust temporal consistency. Superior performance compared to state-of-the-art baselines validates this ability. The model exhibits proficiency when employed for cross-view urban scene generation from satellite images. (性能描述)
论文方法部分
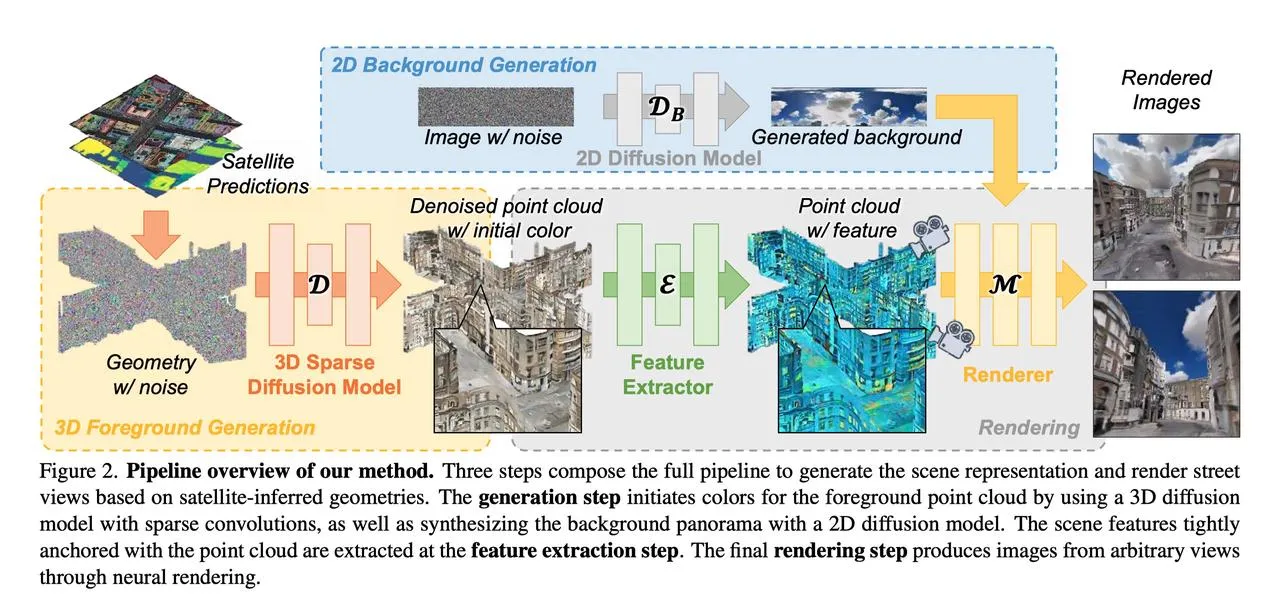
最上面的2D Background Generation比较好理解,使用了2D diffusion模型得到一张背景的全景图,文章中没有提及具体使用了什么diffusion模型。这里的satellite predictions文章中也没有提及具体方式,只是数据集中输入的数据就是“satellite-inferred geometries.”也就是没有颜色的点云。它的3D Sparse Diffusion Model只是给每个建筑外立面点和道路表面点生成了一个颜色(这方面具体公式细节论文也写的不是很清楚,我也没太看懂)。
值得一提的是,在后续的渲染pipeline中,没有直接用得到色彩的点云去做渲染,而是给点云做了个特征提取,将这个特征看做是nerf的编码。原文如下:Instead of fitting a radiance field during test-time optimization, the scene representation (P,F) is obtained in a feed-forward way. 这个M网络就很像nerf中的mlp,会得到渲染光线上采样点的颜色与体密度,最后对颜色与深度都做渲染并计算L1损失。
再次回顾一下这篇论文的方法部分,可以看出,这里的3D foreground generation以及feature extractor部分,个人理解其作用是一个二阶段的编码,相比于ingp的体素化的可学习特征编码,由于这篇文章的任务输入就已经有geometry了,所以直接通过3D diffusion给一个颜色初值,通过一阶段的损失构造得到一个合理的颜色初值(相当于已经有了一个coarse 3d representation了),然后再通过一个特征提取+渲染的方式做二阶段的nerf训练。
由此来看,其实感觉论文标题中的3d urban scene generation其实根本也没做generation(在下面的实验部分更能看出来),from satellite images其实也根本不是从卫星影像出发的,而是用的数据集里就已经有了城市的深度图、全景图、RGBD图像,至于with diffusion则更是有点蹭diffusion的感觉。
论文实验部分
- 使用的数据集
HoliCity and OmniCity,其中HoliCity是6k+ real-world urban scenes in central London,每个场景有high-resolution panorama image, a depth (distance) map, and 8 posed RGB-D pinhole views. 其中OmniCity有satellite images associated with ground-view panoramas但没有street-level geometry。所以作者也没有在OmniCity数据集上做定量指标,而是在这上面跑了卫星图视角的demo去跟MVDiffusion比定性(这很离谱),只在HoliCity数据集上测试了指标,值得一提他的指标是FVD、KVD、FID、KID、PSNR、SSIM、LPIPS、User study,其中FVD、KVD是FID、KID的视频版本,User study是与其他三种方法比较的用户打分
- 对比实验的合理性
个人觉得不太合理
- 训练使用的GPU资源与时间资源,推理速度
不太关注
- 消融实验的合理性,若无消融实验,分析为什么不需要做
有消融实验,但说实话觉得他文章中的消融实验说明不了什么
- 实验中有没有用到一些特殊的技巧
点云平均重采样,由于数据集中用来做geometry的点云分布不是很均匀,因此做了个重采样。
总结
学习一下他的文章行文思路,数据集的选用、baseline的选取、指标的选取、pipeline的设计,都很有技巧。